Michael D. Twardowski1,2, Serge H. Roy1,3, Zhi Li2, Paola Contessa1, Gianluca De Luca1, and Joshua C. Kline1
1Delsys Inc. and Altec Inc., Natick, MA, USA
2Human Inspired Robotics Laboratory, Department of Robotics Engineering,
Worcester Polytechnic Institute, Worcester, MA, USA
3Sargent College of Health & Rehabilitation Sciences at Boston University, Boston, MA, USA
Email: jkline@delsys.com
INTRODUCTION
Modern prostheses have made strident gains in recent years, incorporating electromechanical components that are capable of mimicking human movement to rehabilitate, and restore function for persons living with musculoskeletal impairments. However, effective control of these devices has been hindered by limitations of current human-machine interfaces using amplitude-based myoelectric control schemes prone to variability and delay [1,2]. To address this healthcare need, we developed a human-machine interface, referred to as Motor Unit Drive (MU Drive), to provide control signals that are based on the firing behavior of individual motor units. Motor unit firing rates and recruitment provide natural physiological mechanisms for controlling force and movement in the intact limb [3] and therefore hold promise for more natural control of prostheses.
METHODS
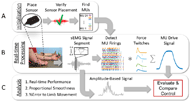
RESULTS
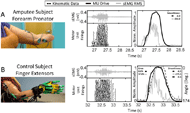
When we compared the MU Drive signal measured from each muscle with that of the amplitude-based signals obtained from the same muscle with a comparable 25 ms delay (i.e., 25 ms window), we observed that MU Drive improves the characteristic smoothness and responsiveness over the myoelectric alternative (Figure 2). Specifically, the MU Drive signal measured from the forearm pronator in the subject with trans-radial amputation during intended forearm pronation/supination maintained a smoothness of -6.0 (Figure 2A, black), a 97.4% improvement relative to the -232.0 smoothness measured from the RMS signal (Figure 2A, gray). Similarly, in the control subject during finger extension/flexion, the MU Drive signal measured from the finger extensors had a smoothness of -6.5 (Figure 2B, black), a 97.6% improvement relative to the -275.5 smoothness measured from the RMS signal (Figure 2B, gray) and closer to the -6.6 smoothness measured from the changes in finger angle of the control subject’s intact limb (Figure 2B, black dashes). When comparing the MU Drive and RMS signals measured from the finger extensors with the changes in joint angle of the 2nd digit of a control subject, we found that MU Drive was able to better replicate changes in movement of the intact limb with a relatively small error of 8.0% (Figure 2B, black), substantially lower than the 57.8% error measured from the RMS signal (Figure 2B, gray).
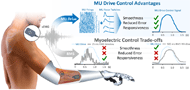
Similarly, we grouped error data across all control subjects for each muscle and movement. The median error of MU Drive was significantly lower (p<0.001) than the measured median error of amplitude-based signals. Importantly we observed the percent error of amplitude-based signals were inversely related to the latency of the response with respect to MU Drive. These data give clear evidence that MU Drive signals are more responsive and more closely replicate the kinematics of the intact limb.
DISCUSSION
These results establish that MU Drive provides noninvasive, real-time access to natural control mechanisms of the human nervous system with significantly improved, smoothness, responsiveness and more faithful replication of the actual or intended limb movement when compared to amplitude-based signals. In fact, our analysis demonstrated amplitude-based signals are limited by an inherent tradeoff: increased smoothness and better replication of actual limb movement requires larger filtering windows that cause increased delay and reduced responsiveness. MU Drive overcomes the trade-off between performance and latency by using direct measures of neural commands that provide a smooth, proportional representation of physiological changes in joint position without the need for computationally expensive filtering. These results persisted across both control and amputee subjects tested, regardless of disuse and changes to muscle morphology due to surgery or congenital formation.
The proof-of-concept demonstrated in this work portrays significant advantages of MU Drive over conventional myoelectric human-machine interfaces. The improved performance characteristics of MU Drive are supported by the use of high-fidelity noninvasive sensors and real-time recognition algorithms to provide noninvasive access to commands of the nervous system for improving control assistive and prosthetic devices.
IMPLICATIONS
This work provides a foundation for future development of motor-unit-based controllers that leverage multiple MU Drive signals across synergistic muscles to enable simultaneous multi-degree-of-freedom control. The technology holds promise for improving assistive device function by achieving advanced control that better reflects user intent. It may also provide a new alternative for advancing the control of exoskeletons, and other rehabilitation applications.
REFERENCES
[1] Guanglin L., Schultz, A., Kuiken, T. Quantifying pattern recognition—based myoelectric control of multifunctional transradial prostheses. IEEE Transactions on Neural Systems and Rehabilitation Engineering. 2010 18(2):185-192.
[2] Schultz, A., Kuiken, T. Neural interfaces for control of upper limb prostheses: the state of the art and future possibilities. Physical Medicine & Rehabilitation. 2011 3(1):55-67.
[3] De Luca, C. J., Erim, Z. Common drive of motor units in regulation of muscle force. Trends in Neurosciences, 1994 17(7): 299–305.
ACKNOWLEDGEMENTS
This research was supported by the De Luca Foundation, Delsys Inc., and by grants from the National Institute of Neurological Disorders and Stroke (R43NS093651) and the Eunice Kennedy Shriver National Institute of Child Health and Human Development (R44HD094626) of the National Institutes of Health.