Joseph A. Majdi1
, Parag V. Chitnis
1
, Siddhartha Sikdar
1
1
George Mason University
INTRODUCTION
A significant challenge in improving the design of assistive technologies for rehabilitation of individuals with neuromuscular deficits is the limited ability to robustly sense muscle activity and function. For the past 50 years, surface electromyography (sEMG) has been the dominant standard for detecting muscle activity for prosthetics[1] and is also widely used for rehabilitation and biomechanics research. However, sEMG lacks spatial specificity and suffers from poor SNR[2]. Despite these limitations, sEMG has been widely used for estimating muscle force, and fatigue[3]. However, sEMG is generally incompatible with electrical stimulation, which is often used either as a therapeutic intervention or in conjunction with assistive technologies, as sEMG signals are typically saturated by current injection during electrical stimulation.
Due to the well-documented limitations of sEMG, alternative biosignal sensing methods for studying muscle activation have been investigated. Mechanomyography (MMG) is a class of mechanical alternatives to sEMG, which record forces and physical energy transfer from muscle activation. Several variants exist from measuring muscle radial displacement (tensiomyography, TMG)[4] to passively recording soundwaves generated by muscle contractions (acoustic myography, AMG)[5,6]. Because these techniques measure mechanical activity (as opposed to electrical activity), they provide a meaningful measure of active muscle contractions that is not dependent on excitation-activation coupling[5]. Further, they would be significantly less susceptible electrical aberrations induced by FES current injection. While these methods have some advantages over sEMG, they suffer from their own limitations, including susceptibility to motion artifacts, and the limited sensitivity to deep-seated muscle motion[7].
Recently, the use of ultrasound imaging has gathered more attention in research for numerous applications to detect and quantify muscle activity. These have included estimating muscle force generation using muscle fiber pennation angle, cross sectional area, and tissue motion tracking[8–13], detecting passive and active muscle activation using fast M-mode or TDI approaches[14,15], and using ultrasound-derived signals for controlling prosthetics[16–18]. A major challenge with ultrasound systems have been the bulky form factor of the transducers and instrumentation, which is a significant limitation to deploying in a wearable system during free unconstrained movement. In this paper, we investigated the use of dual-element continuous-wave (CW) Doppler ultrasound systems, which can be deployed in a small form factor for wearable use alongside assistive devices. To demonstrate the value of this method, we investigated its utility in estimating muscle force production and fatigue during electrical stimulation, a situation where conventional sEMG cannot be utilized.
METHODS
Experimental setup
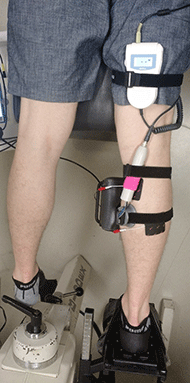
Six healthy male subjects were recruited for the experiments under a protocol approved by the George Mason University Institutional Review Board. Subjects were asked to lie in a prone position, and the isometric plantar flexion torque was measured (Fig 1) using a Biodex II dynamometer upgraded with a Humac interface (CSMi Solutions) interfaced with LabView (National Instruments). Subjects were instrumented with a modified BT-200 Hi-Dop vascular continuous wave Doppler ultrasound probe (Bistos), placed over the muscle body of the medial head of the gastrocnemius. Care was taken to place the probe away from blood vessels so that the movement detected by the probe was from muscle/tissue motion alone. The probe was also shielded in a grounded aluminum enclosure to minimize electromagnetic interference (not shown). Rehastim2 (Hasomed) ES electrodes were placed on the proximal part of the medial head and distal part of the lateral head of the gastrocnemius in accordance with the Rehastim2 manual. ES was controlled with a custom Matlab/Simulink (MathWorks) interface.
Experimental protocol
First, subjects were asked to perform voluntary plantar flexion, and the maximum voluntary isometric torque (MVIT) was recorded. We then applied 50Hz, 100µs biphasic current at progressively higher amperage, and the current required to produce approximately 20% of their MVIT was recorded as the stimulation current. A stimulation cycle of 1 second duration was designed as follows: The current during the on phase was ramped up from zero to maximum over 0.12s, held at maximum current for 0.5s, and then ramped down to zero for 0.12s, followed by 0.26s rest, with a total stimulation period of 1s. We then stimulated the calf for 60 cycles over a period of 60 seconds. The peak torque declined significantly to below 50% of the initial value for all subjects during this 60 second stimulation, indicating the onset of fatigue. The muscle was then allowed to rest for two minutes, and the 60-second stimulation cycles was repeated for a total of five trials. Audio output from the CW Doppler instrument was recorded continuously throughout the entire experiment.
Analysis
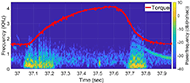
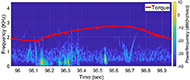
The muscle contraction velocity was estimated using the CW Doppler signal. We calculated the spectrogram (Fig 2) and spectral entropy of the CW Doppler signal to analyze signal duration at the onset of muscle contraction. The CW Doppler instrument had a built in high-pass clutter filter with a cutoff of 500Hz to suppress slow motion. On occasion, multiple activation signals were present (presumably from the gastrocnemius and soleus activating at different recruitment times). In these cases, only the first signal was analyzed. We compared the signal duration first and 60th stimulation period and performed a simple linear regression between the signal duration and peak plantar flexion torque for stimulation cycles 1, 11, 21, 31, 41, 51, and 60 for each of the five ES trials.
RESULTS
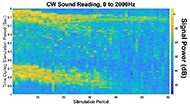
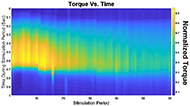
We found that CW Doppler signals at both the onset phase and release phase of force, were force/fatigue dependent (Fig 3). In general, as the muscles fatigued, the audio signal durations continually got shorter during both phases, and tended to drop below detectable levels for the release phase.
For the first stimulation period, the contraction signal was on average 133.5ms ± 36.05ms SD. By the 60th stimulation period, the muscle had fatigued and the signal duration dropped to 52.16ms ± 20.24ms. This change was statistically significant (p = 0.012, paired 2-tailed t-test). This indicates that the signal duration is correlated with peak plantar flexion torque.
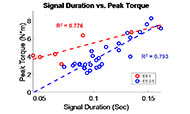
DISCUSSION
Our results indicate that CW Doppler can be utilized in conjunction with electrical stimulation to infer the muscle force production as well as the onset of fatigue. This method can overcome a major limitation of sEMG, which cannot be utilized in conjunction with ES.
CW Doppler can be performed with a dual-element transducer with a small footprint, and miniaturized battery-operated electronics, making this technology well-suited for a wearable application. CW Doppler can be sampled at relatively low sample rate (44-100kHz, audio range) and can be analyzed with fast Fourier transform algorithms, which makes real-time analysis possible. We found that the duration of the CW Doppler signal after a high-pass clutter filter, correlated with peak muscle force, throughout five trials of ES stimulation, fatigue, and recovery. One interpretation of our data is that the muscle shortening velocity decreases as the muscle fatigues, and the measured signal duration that exceeds the clutter filter cutoff of 500Hz decreases with fatigue.
The use of CW Doppler to measure muscle contraction velocities has a significant advantage over conventional ultrasound imaging methods that rely on measurement of muscle architecture features such as pennation angle and cross-sectional area. Image-based measures are highly sensitive to transducer positioning, and out of plane movement that is common during muscle contraction.
In this study, we utilized a commercial vascular CW probe to demonstrate the feasibility of this approach. The use of a commercial system has limitations. First, this system was designed to measure blood flow and hence has a clutter filter designed to block signal from low velocity tissue movement. However, the Doppler shift due to muscle contractions were sufficiently large to exceed the clutter filter settings. Doppler signals for lower velocities, such as those produced during the relaxation phases can sometimes fall below the clutter filter cutoff, especially after muscle fatigue. Additionally, because we are measuring the audio output alone, we lose the phase and thus directionality of the movement, and instead are left with only magnitude. In future work, we plan to bypass the internal signal processing circuitry and record the in phase and quadrature (IQ) components to both preserve directionality and bypass the clutter filter to make slower tissue movements detectable. A second limitation is that CW Doppler does not provide depth-specific information. We are investigating the use of frequency-encoded methods that can enable depth-specific velocities without significantly increasing the form factor of the transducer and associated instrumentation.
CONCLUSIONS
Continuous wave Doppler ultrasound is portable, low power, and provides real time information about muscle activation, fatigue, and recovery that is compatible with ES. This could provide a useful wearable biosignal sensing method for use in conjunction with assistive technologies such as hybrid exoskeletons combining ES and actuators, and therapeutic electrical stimulation. This method overcomes many of the limitations of other sensing modalities.
REFERENCES
- Ravindra V, Castellini C. A Comparative Analysis of Three Non-Invasive Human-Machine Interfaces for the Disabled. Front Neurorobotics [Internet]. 2014 [cited 2017 Oct 3];8. Available from: https://www.frontiersin.org/articles/10.3389/fnbot.2014.00024/full
- Akhlaghi N, Baker CA, Lahlou M, Zafar H, Murthy KG, Rangwala HS, et al. Real-Time Classification of Hand Motions Using Ultrasound Imaging of Forearm Muscles. IEEE Trans Biomed Eng. 2016 Aug;63(8):1687–98.
- Zhang Q, Hayashibe M, Fraisse P, Guiraud D. FES-Induced Torque Prediction With Evoked EMG Sensing for Muscle Fatigue Tracking. IEEEASME Trans Mechatron. 2011 Oct;16(5):816–26.
- Macgregor LJ, Hunter AM, Orizio C, Fairweather MM, Ditroilo M. Assessment of Skeletal Muscle Contractile Properties by Radial Displacement: The Case for Tensiomyography. Sports Med. 2018 Jul 1;48(7):1607–20.
- Barry DT, Geiringer SR, Ball RD. Acoustic myography: A noninvasive monitor of motor unit fatigue. Muscle Nerve. 1985 Mar;8(3):189–94.
- Harrison AP, Danneskiold-Samsøe B, Bartels EM. Portable acoustic myography - a realistic noninvasive method for assessment of muscle activity and coordination in human subjects in most home and sports settings. Physiol Rep [Internet]. 2013 Jul [cited 2019 Jan 12];1(2). Available from: http://doi.wiley.com/10.1002/phy2.29
- Castellini C. Design Principles of a Light, Wearable Upper Limb Interface for Prosthetics and Teleoperation. In: Wearable Robotics [Internet]. Elsevier; 2020 [cited 2020 Feb 27]. p. 377–91. Available from: https://linkinghub.elsevier.com/retrieve/pii/B9780128146590000205
- Lieber RL, Friden J. Functional and clinical significance of skeletal muscle architecture. Muscle Nerve. 2000 Nov;23(11):1647–66.
- Ito M, Kawakami Y, Ichinose Y, Fukashiro S, Fukunaga T. Nonisometric behavior of fascicles during isometric contractions of a human muscle. J Appl Physiol. 1998 Oct;85(4):1230–5.
- Bakke M, Tuxetv A, Vilmann P, Jensen BR, Vilmann A, Toft M. Ultrasound image of human masseter muscle related to bite force, electromyography, facial morphology, and occlusal factors. Eur J Oral Sci. 1992 Jun 1;100(3):164–71.
- Witte RS, Dow DE, Olafsson R, Shi Y, O'Donnell M. High resolution ultrasound imaging of skeletal muscle dynamics and effects of fatigue. In: IEEE Ultrasonics Symposium, 2004 [Internet]. Montreal, Canada: IEEE; 2004 [cited 2018 Sep 5]. p. 764–7. Available from: http://ieeexplore.ieee.org/document/1417834/
- Witte RS, Kim K, Martin BJ, O'Donnell M. Effect of Fatigue on Muscle Elasticity in the Human Forearm Using Ultrasound Strain Imaging. In: Conf Proc IEEE Eng Med Biol Soc [Internet]. IEEE; 2006 [cited 2018 Mar 17]. p. 4490–3. Available from: http://ieeexplore.ieee.org/lpdocs/epic03/wrapper.htm?arnumber=4398449
- Stavdahl O, Gronningsaeter A, Malvig KE. ULTRASOUND BASED ESTIMATION OF MUSCLE CONTRACTION. Myoelectric Symp. 1997;7.
- Pulkovski N, Schenk P, Maffiuletti NA, Mannion AF. Tissue Doppler imaging for detecting onset of muscle activity. Muscle Nerve. 2008 May;37(5):638–49.
- Dieterich AV, Botter A, Vieira TM, Peolsson A, Petzke F, Davey P, et al. Spatial variation and inconsistency between estimates of onset of muscle activation from EMG and ultrasound. Sci Rep [Internet]. 2017 Dec [cited 2018 Dec 5];7(1). Available from: http://www.nature.com/articles/srep42011
- Sikdar S, Rangwala H, Eastlake EB, Hunt IA, Nelson AJ, Devanathan J, et al. Novel Method for Predicting Dexterous Individual Finger Movements by Imaging Muscle Activity Using a Wearable Ultrasonic System. IEEE Trans Neural Syst Rehabil Eng. 2014 Jan;22(1):69–76.
- Castellini C, Passig G, Zarka E. Using Ultrasound Images of the Forearm to Predict Finger Positions. IEEE Trans Neural Syst Rehabil Eng. 2012 Nov;20(6):788–97.
- Shi J, Guo J-Y, Hu S-X, Zheng Y-P. Recognition of Finger Flexion Motion from Ultrasound Image: A Feasibility Study. Ultrasound Med Biol. 2012 Oct;38(10):1695–704.
ACKNOWLEDGEMENTS
This work was supported by an NSF Cyberphysical Systems Grant, number CNS 1646204.