Zijian Huang1,2
, Yousif Shwetar
1,3
, Akhila Veerubhotla
1,2
, Dan Ding
1-3
1
Human Engineering Research Laboratories, Department of Veterans Affairs,
2
Department of Rehabilitation and Science Technology, University of Pittsburgh,
3
Department of Bioengineering, Swanson School of Engineering, University of Pittsburgh
INTRODUCTION
There are an estimated 291,000 people with Spinal Cord Injury (SCI) living in the United States [1]. Many individuals with SCI rely on wheelchairs for mobility [2]. Despite the convenience brought by wheelchairs, these individuals are less physically active when compared with able-bodied persons [3]. While sufficient physical activity (PA) has been linked with improved functional capacity and reduced risks of secondary conditions in this population [4], PA promotion efforts are often not given enough attention in the traditional rehabilitation [5]. Quantifying free-living PA is an essential part of PA promotion, particularly in tracking the time spent at different intensity levels as recommended by the PA guidelines [6]. Traditional self-report questionnaires are burdensome and subject to recall bias. With the proliferation and cost reduction of wearable devices, using such devices to track habitual PA becomes a popular approach nowadays [7].
Among all commercially available wearable devices, ActiGraph activity monitors (ActiGraph, Inc., FL, USA) are the most frequently used devices by researchers, accounting for over 50% of published studies [8]. Among all ActiGraph devices, the GT3X model and GT9K Link model are the two most used triaxial accelerometer-based devices that can not only record raw accelerometer signals at a set frequency but also generate a proprietary variable named 'counts' via its software ActiLife. Although the algorithms used to convert raw acceleration signals to 'counts' are not publicly available, 'counts' are widely adopted today in research communities, as they are perceived easy to use and easy to interpret with the quantity indicating the movement intensity. Also, no specific expertise on signal processing, such as rectification and filtering are needed to use 'counts' as compared with handling raw acceleration signals [9]. A few studies have established threshold 'counts' for distinguishing different activity intensities in manual wheelchair users (MWUs) with SCI [10-12]. In addition, a number of studies developed custom energy expenditure predictive equations based on 'counts' for MWUs [13-17]. As all these studies used the proprietary 'counts' in their predictive equations, their derived thresholds and custom equations can only be used by those who have access to ActiGraph devices along with its costly software. With the performance improvement and price drop of smartwatches and fitness bands, it would be helpful to equip these devices with the same capabilities to classify activity intensities and predict energy expenditure for MWUs. Brønd et al. proposed a method that utilized a series of filtering, sampling, and signal processing techniques to convert raw acceleration signals to 'counts', and evaluated if the 'counts' generated using their custom method match the ActiGraph 'counts' for a number of ambulatory activities [18]. Their result showed that there is a small percentage bias of 2.2% between generated 'counts' and the ActiGraph proprietary 'counts' under the setting of hip-worn 24 hours free-living condition.
In this study, we followed Brønd et al.'s approach to convert raw acceleration signals to 'counts', and evaluated if the 'counts' generated using this custom method match the ActiGraph 'counts' over a wide range of PA performed by MWUs with SCI who worn an ActiGraph device on the dominant wrist. If the equivalence between the two measures is established, the predictive equations or thresholds developed based on ActiGraph 'counts' for MWUs with SCI could be used by other wearable devices that record raw accelerometer signals, greatly expanding the range of devices available for MWUs as well as professionals who work with this population.
METHODS
This study was conducted at the Human Engineering Research Laboratories (HERL), Pittsburgh, and the James J Peters VA Medical Center, Bronx. The study was approved by the US Department of Veterans Affairs's Central Institutional Review Board. The inclusion criteria for this study is: 1) having a spinal cord injury, 2) between 18 and 65 years, 3) using a manual wheelchair as their primary means of mobility (≥40 hours/week), 4) at least one-year post injury, and 5) medically stable.
Study protocol:
After informed consent, demographics and anthropology data were collected. Participants then rested in a supine position for 20 minutes to acquire resting metabolic data and then randomly performed a series of activities of daily living (ADLs) and exercises for 10 minutes with a minimum 3-minutes break between each activity. The activities included: propulsion at self-selected slow, normal, and fast pace on flat tiled surface; propulsion up/down a slope; watching TV; working on a computer; practicing shooting a basketball; sweeping/vacuuming the floor; loading and unloading a dishwasher; weight lifting; TheraBand exercises; arm ergometry exercise at a self-selected slow and fast pace; folding laundry; and being pushed in their wheelchair. All activities were video recorded.
An ActiGraph GT9X Link was used to capture upper limb movements. Subjects were instructed to wear the ActiGraph device on the dominant wrist. The 60-sec epochs 'counts', as well as the raw accelerometer signals sampled at 30Hz, were obtained using the ActiLife software v6.11.9. Subjects also wore a COSMED K4b2 (COSMED, srl., Rome, Italy) portable metabolic cart during all activity trials which collects oxygen consumption (VO2) and carbon dioxide output (VCO2). For each activity, VO2 values (ml/min) were averaged for each minute and divided by the product of resting metabolic equivalent of 2.7 (ml/kg/min) and participant's weight (kg) to obtain the Metabolic Equivalent of Task (MET) for that minute[12]. The ActiGraph device and the K4b2 metabolic cart were calibrated following standard procedures and time-synchronized [19-20]. All activities were coded and annotated with the synchronized timestamp in the dataset.
Data preparation:
Characteristics | M | SD |
---|---|---|
Age (years) | 40 | 13 |
Height (inches) | 69 | 4 |
Weight (kg) | 83 | 21 |
M | % | |
Gender | ||
Male | 26 | 81 |
Female | 6 | 19 |
Lesion Level | ||
Cervical | 2 | 6 |
Thoracic | 26 | 82 |
Lumbar | 2 | 6 |
Not Reported | 2 | 6 |
Lesion Type | ||
Complete | 18 | 56 |
Incomplete | 8 | 25 |
Not Reported | 6 | 19 |
The following data preparation rules were applied to clean up the data: 1) any data without an activity annotation was removed; 2) if either K4b2 or ActiGraph data was not available for any 60-second epoch, the epoch was dropped. The 'counts' generation method proposed by Brønd et al.[17] first removes noise and artifacts from raw signals using anti-aliasing and band pass filter. The signals are then truncated with 2.13g constant amplitude and rectified. Finally, the signals are applied with a dead-band threshold of 0.068g, converted to 8-bit resolution and integrated into 1-second epochs. Python script from Brønd's GitHub repository was used to generate the 'counts' from the raw accelerometer signals.
Data analysis:
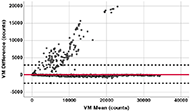
A one sample t-test was used to determine if there is a significant difference between 'counts' generated by Brønd et al.'s approach and 'counts' output by the ActiGraph device. A Bland-Altman plot of all activity data from all subjects was used to visualize and assess the bias between the two 'counts'. A simple linear regression of the difference and the mean was calculated to assess the relation between the bias and intensity levels. Finally, a regression-based equivalence test was performed to directly quantify the degree of agreement between the two 'counts', where a regression model was fitted to the pairs of ActiGraph 'counts' and 'counts' by Brønd et al.'s approach. If the two 'counts' are equivalent, the intercept of this regression model should be 0 and the slope should be 1. We defined the equivalence in this project as ±5% between the two 'counts', which means the slope should be between 0.95 and 1.05 and the intercept should be ±5% of the mean ActiGraph 'counts'. To claim equivalence between the two 'counts' at a=0.05, the 90% confidence interval (CI) for the intercept and the slope should fall inside their respective equivalence region [21]. All data analysis is performed by SPSS version 26.
In addition, to demonstrate if the two 'counts' yield consistent results when they ae used to classify activity intensities, we applied the 'counts' thresholds developed by Veerubhotla et al. to both 'counts' across all activities. The threshold between sedentary and light intensity was 2,057 'counts' per minute (CPM), and between light and moderate to vigorous intensity physical activities (MVPA) was 11,551 CPM [12].
RESULTS
A total of 32 participants completed this study. Demographic data is shown in Table 1. A total of 4,769 minutes of data was selected after data preparation. The one-sample t-test indicated that the difference (Mean = 99.6, SD = 1,418, 95% CI [59.3, 139.9]) between the ActiGraph 'counts' (Mean = 9,143, SD = 7,149, Median = 8,554, 95% CI
Activity intensity | ActiGraph | Generated | Difference (%) |
---|---|---|---|
Sedentary | 1032 | 1043 | 11 (1.1) |
Light | 2234 | 2225 | 9 (0.4) |
MVPA | 1503 | 1501 | 2 (0.1) |
[8,940, 9,346]) and the generated 'counts' (Mean = 9,044, SD = 7,133, Median = 8,432, 95% CI [8,841, 9,246]) is larger than 0, t(4768) = 4.85, p < .001. The mean percentage difference is 0.95% ± 15.01% with 95% CI [0.51%-1.39%]. Figure 1 shows the Bland-Altman plot with x-axis being the average of the two 'counts' and y-axis being the difference between the two 'counts'. The plot showed the mean bias between the two counts is 99.6, and the limits of agreement were −2,680 and 2,880. No significant linear regression relationship was found between the bias and the activity level (F(1,4767) = 0.580, p = .446), with an R2 of 0. In terms of the equivalence testing, the mean of the ActiGraph 'counts' is 9,143, meaning the ±5% equivalence region is (-457, 457) for the intercept, and (0.95, 1.05) for the slope. The simple linear regression between the Actigraph 'counts' and the generated 'counts' yielded a significant linear relationship with estimated slope of 0.978, 90% CI [0.973, 0.983] and intercept of 100.2, 90% CI [45.7, 154.7]. Both the slope and intercept fell inside their respective 5% equivalence region. Table 2 showed the classification result based on the CPM threshold using both 'counts', with the classification difference ranging from 0.1% for MVPA and 1.1% for sedentary time.
DISCUSSION
In this study, we followed Brønd et al.'s approach to convert raw acceleration signals into 'counts'. While Brønd et al. validated this approach in an ambulatory group with a hip-worn device, we extended this validation to a wide range of wheelchair related activities performed by 32 MWUs with SCI who wore the device around their dominant wrist. Although the mean difference between the generated 'counts' and the Actigraph proprietary 'counts' significantly differed from 0, the mean difference of 99.6 is relatively small, considering that the mean Actigraph 'counts' being 9,143. The mean percentage bias of 0.95% is also smaller than what was reported in Brønd et al.'s study (2.2%). The equivalence testing further proved that the two measures are statistically equivalent within 5%. Furthermore, when using both 'counts' to classify time spent in sedentary, light, and MVPA, the yielded differences are quite small, ranging from 0.1% for MVPA and 1.1% for sedentary time. However, when examining the Bland-Altman plot, while most of the bias fell near 0, there are a number of minutes (2.64% with the differences beyond the upper and lower limits of agreement) that had quite large differences between the two 'counts'. It was not clear yet what factors may have caused the large discrepancies for this small percent of minutes.
It is noticeable that our mean bias between the two 'counts' is larger than what was reported in Brønd et al.'s study. This could be due to the different wearing position (wrist vs hip) and trial setting (type and range of activities). The device worn at the wrist is expected to register small movements associated with many activities while the hip location is only sensitive to ambulatory activities. Our participants were instructed to perform a wide range of PA and exercises during the lab trial, while Brønd et al.'s study was carried out in 24h free-living setting, which may have a higher percent of sedentary time.
Our results showed low difference and high equivalence between the generated 'counts' and the ActiGraph 'counts', indicating that thresholds, algorithms, and models that developed based on ActiGraph's proprietary 'counts' can be applied to 'counts' generated from raw accelerometer signals without retraining or redeveloping. This means researchers and clinical professionals could access these thresholds, algorithms, and models via other cheaper commercial devices that can record raw acceleration signals. The 'counts'-based algorism could be integrated into these devices to improve their ability to predict energy expenditure and activity intensity for MWUs and help these individuals to self-manage their PA.
CONCLUSION
This study converted raw acceleration signals to ActiGraph 'counts' for a variety of wheelchair-related activities and compared it with the criterion proprietary 'counts'. High equivalence, low classification difference, and low errors were confirmed between the two measures, indicating the feasibility for other wearable devices to adopt established thresholds, equations, and models based on ActiGraph proprietary 'counts'.
REFERENCES
- National Spinal Cord Injury Statistical Center, Facts and Figures at a Glance. Birmingham, AL: University of Alabama at Birmingham, 2019 https://www.nscisc.uab.edu/Public/Facts%20and%20Figures%202019%20-%20Final.pdf
- Chaves, E. S., Boninger, M. L., Cooper, R., Fitzgerald, S. G., Gray, D. B., & Cooper, R. A. (2004). Assessing the influence of wheelchair technology on perception of participation in spinal cord injury. Archives of physical medicine and rehabilitation, 85(11), 1854-1858.
- Kehn, M., & Kroll, T. (2009). Staying physically active after spinal cord injury: a qualitative exploration of barriers and facilitators to exercise participation. BMC Public Health, 9(1), 168.
- World Health Organization. (2007). A European framework to promote physical activity for health. Geneva: WHO.
- Weaver, F. M., & LaVela, S. L. (2007). Preventive care in spinal cord injuries and disorders: examples of research and implementation. Physical medicine and rehabilitation clinics of North America, 18(2), 297-316.
- CDC. Adults with Disabilities -- vital signs for disease control and prevention factsheet 2014. May 6, 2014; http://www.cdc.gov/vitalsigns/disabilities/. Accessed 9/20/2016.
- Ridgers, N. D., McNarry, M. A., & Mackintosh, K. A. (2016). Feasibility and effectiveness of using wearable activity trackers in youth: a systematic review. JMIR mHealth and uHealth, 4(4), e129.
- Migueles, J. H., Cadenas-Sanchez, C., Ekelund, U., Nyström, C. D., Mora-Gonzalez, J., Löf, M., ... & Ortega, F. B. (2017). Accelerometer data collection and processing criteria to assess physical activity and other outcomes: a systematic review and practical considerations. Sports medicine, 47(9), 1821-1845.
- Ridgers, N. D., McNarry, M. A., & Mackintosh, K. A. (2016). Feasibility and effectiveness of using wearable activity trackers in youth: a systematic review. JMIR mHealth and uHealth, 4(4), e129.
- Learmonth YC, Kinnett-Hopkins D, Rice IM, Dysterheft JL, Motl RW. Accelerometer output and its association with energy expenditure during manual wheelchair propulsion. Spinal cord. 2016;54(2):110-4.
- McCracken LA, Ma JK, Voss C, Chan FH, Martin Ginis KA, West CR. Wrist Accelerometry for Physical Activity Measurement in Individuals With Spinal Cord Injury-A Need for Individually Calibrated Cut-Points. Archives of physical medicine and rehabilitation. 2018;99(4):684-9.
- Veerubhotla, A. (2019). How Well Can Wearable Devices Measure Physical Activity in Manual Wheelchair Users with Spinal Cord Injury? (Unpublished doctoral dissertation). University of Pittsburgh, PA.
- Learmonth YC, Kinnett-Hopkins D, Rice IM, Dysterheft JL, Motl RW. Accelerometer output and its association with energy expenditure during manual wheelchair propulsion. Spinal cord. 2016;54(2):110-4.
- Tsang K. Using wearable sensors for physical activity measurement and promotion in manual wheelchair users [Dissertation]. Pittsburgh (PA): University of Pittsburgh; 2018.
- Garcia-Masso X, Serra-Ano P, Garcia-Raffi LM, Sanchez-Perez EA, Lopez-Pascual J, Gonzalez LM. Validation of the use of actigraph GT3X accelerometers to estimate energy expenditure in full time manual wheelchair users with spinal cord injury. Spinal Cord. 2013;51:898–903.
- Nightingale TE, Walhim JP, Thompson D, Bilzon JL. Predicting physical activity energy expenditure in manual wheelchair users. Med Sci Sports Exerc. 2014;46:1849–58.
- Nightingale TE, Walhin JP, Thompson D, Bilzon JL. Influence of accelerometer type and placement on physical activity energy expenditure prediction in manual wheelchair users. PLoS ONE. 2015;10:e0126086.
- Brønd, J. C., Andersen, L. B., & Arvidsson, D. (2017). Generating ActiGraph counts from raw acceleration recorded by an alternative monitor.
- Sandroff, B. M., Motl, R. W., & Suh, Y. (2012). Accelerometer output and its association with energy expenditure in persons with multiple sclerosis. Journal of Rehabilitation Research and Development, 49(3), 467.
- Pinnington, H. C., Wong, P., Tay, J., Green, D., & Dawson, B. (2001). The level of accuracy and agreement in measures of FEO2, FECO2 and VE between the Cosmed K4b2 portable, respiratory gas analysis system and a metabolic cart. Journal of Science and Medicine in Sport, 4(3), 324-335.
- Dixon, P. M., Saint-Maurice, P. F., Kim, Y., Hibbing, P., Bai, Y., & Welk, G. J. (2018). A primer on the use of equivalence testing for evaluating measurement agreement. Medicine and science in sports and exercise, 50(4), 837.
ACKNOWLEDGMENTS
This study is funded by the VA Rehabilitation Research & Development, Merit Review grant #1I01RX000971- 01A2. It is being conducted at the Human Engineering Research Laboratories (Pittsburgh, PA). The contents do not represent the views of the Department of Veterans Affairs or the United States Government.