Gabriel Diamond-Ouellette1, Laurent Bouyer1,2, Krista Best1,2
1Centre for Interdisciplinary Research in Rehabilitation and Social Integration (CIRRIS), Quebec City, Canada, 2Université Laval, Quebec City, Canada
INTRODUCTION
Walking is a common mode of transportation used in various occupational environments. In many workplaces, individuals are required to carry heavy loads on their back, which can alter gait patterns. Optimizing gait patterns has been shown to enhance physiological responses, including the metabolic cost of walking (MCW) [1,2]. However, load carriage during walking (e.g., backpacks for hiking, fragmentation vests for military or law enforcement tasks), alters gait biomechanics and disrupts normal physiological responses [3]. Moreover, altered gait patterns due to heavy load carriage may increase the risk of acute injuries, which overtime may lead to repetitive strain injuries [4].
Exoskeletons, defined as wearable devices that can augment, facilitate, assist, or enhance motion, posture, or physical activity [5], represent a novel technology that may support load carriage to reduce metabolic costs and risk of injury during walking. Research on active (I.e., requiring a power source) and passive (i.e., no external power) exoskeletons has shown that reductions in the MCW is possible [6–8], but that some full body or quasi-passive exoskeletons may increase the MCW. Results from research on exoskeleton during load carriages over treadmill observed a 60% increase in VO2 [9] or 36% in energy expenditure when walking on a treadmill at 1.11 m/s [10]. The results of these studies suggested that participants lacked adequate familiarization, stating that the limited effect of the exoskeleton was likely due to a learning effect and that participants did not have enough time to adapt to walking with the exoskeleton [10].
Familiarization with novel technologies is important for safe and effective user uptake [11]. A study on customized passive load-bearing exoskeletons exploring the influence of a familiarization period observed that a reduction in MCW was possible if the principles of motor learning were adequately integrated [7]. Another recent study highlighted the need to ensure adequate exoskeleton training for participants, using longer experimental protocols that support adequate time for familiarization [12]. Evidence supports the ability to adapt to using various technologies, but recommended exposure times vary depending on multiple factors, such as the complexity of the exoskeleton and previous user experience. Although the fit of the exoskeleton to the user was shown to be a major factor predicting performance and acceptability of the device [13], fitting of the exoskeleton has rarely been documented.
Exoskeletons that are customized to user anthropometry and perceived comfort may offer superior compatibility for the human and limit the drawbacks of purely mechanical concepts. However, customizable exoskeleton limits wide-range accessibility to various users due to the customization of mechanical parts, complexity of adjustment and costs. Adjustable exoskeleton may overcome some of the barriers to accessing customized devices; however, optimal physiological responses and performance may be compromised.
The objectives of this study were to 1) explore the differences between the UPRISE (Ultralight Passive Ruggedized Integrated Soldier Exoskeleton) customized exoskeleton (Gen 3.0) and an UPRISE adjustable exoskeleton (Gen 4.0) on MCW and 2) how the familiarization will influence the MCW. It was hypothesized that the Gen 3.0 exoskeletons would provide better benefit than the Gen 4.0, but the familiarization would affect MCW similarly.
METHODS
Participants
Two datasets were combined for this study. The first dataset included a purposive sample of three male soldiers who were recruited from the Canadian Army Infantry while the second dataset included a convenient sample of three healthy participants. To be eligible, participants had: 1) to be older than 18 years old without injuries limiting load carriage and walking; 2) being naïve to wearing an exoskeleton; 3) self-report at least "moderately active" or "active" on the Godin Leisure- Time Exercise Questionnaire for the healthy participant or to have successfully completed the Canadian Armed Forces (CAF) physical employment standard test.
Exoskeleton
Customized exoskeleton
The customized passive load-bearing exoskeleton prototype (UPRISE Gen 3.0), weighing on average 7.2 ± 1.1 kg was developed to provide load transfer capacity and was custom-fit to each soldier. The custom fitting process was a multiple steps that included a first session with the participants to collect anthropometric measurements via anatomical markers and a 3D scan of the whole body.
Adjustable exoskeleton
The adjustable exoskeleton prototype (UPRISE Gen 4.0), weighing on average 10 kg also provide load transfer capacity, but could be adjusted to multiple participants with change in exoskeleton parts. The process of fitting the exoskeleton was by taking measurements of the participant (e.g., thigh length and circumference, etc.) in order to select the exoskeleton parts appropriate for that participant. The exoskeleton was then assembled and fitted on the participant in order to align the joint correctly. The participant was allowed to do minimal movements in order to provide feedback on mobility and comfort before starting the experimental tests.
Familiarization
Two familiarization periods were developed for the project and were based on the principle of motor learning. Participants wearing the exoskeleton Gen 3.0 had a familiarization period that consisted of three-hour daily sessions for 9 days distributed over two consecutive weeks while Gen 4.0 participants had a total of 14 days of training (around 1h30) distributed over a period of four weeks. The familiarization period was almost the same between dataset, but as participants of Gen 3.0 were under a tight schedule, the distribution of practice principle was omitted. Pertinent principles of motor learning were included in both familiarization periods (i.e., progression, variability in tasks, feedback, etc.).
Data collection
Testing included three conditions: 1) a control condition with no exoskeleton (NoExo); 2) the first exposure to the exoskeleton (ExoPre) and; 3) after a period of familiarization with the exoskeleton (ExoPost). Participants walked at eight (1.8, 2.4, 3.0, 3.6, 4.2, 4.8, 5.4, 6.0 km/h) for the Gen 3.0 and six preselected speeds (1.5, 1.9, 2.3, 2.7, 3.1, 3.5 mph) for the Gen 4.0 exoskeletons. The order of speeds was randomized for each testing condition.
For each testing session, participants were instructed to: 1) wear the equipment (Gen 3.0 = 38 kg, Gen 4.0 = 22.75 kg) and the exoskeleton if applied; 2) remain still for 10 minutes to collect VO2 at rest without the effect of the added weight on them (VO2 rest); 3) walk on a motorized treadmill until they reached a steady state Vo2 (VO2 SS; at least 10 min); and 4) rest sitting on a chair with the equipment removed until VO2 rest returned (approx. 15 min). This sequence was repeated for each of the walking speeds.
Breath-by-breath O2 and CO2 measures were collected during the whole testing protocol using a portable spiroergometry gas exchange system (Gen 3.0 = Metamax® 3b, Cortex, Leipzig, Germany, Gen 4.0 = K5, COSMED, Rome, Italy). The analyzers were calibrated according to the manufacturer's instruction.
Data processing
The net MCW was estimated using the difference between the measured rate of oxygen exchange (VO2) during rest and steady-state walking (last two minutes of each standing and walking trial). The VO2 rate was first normalized to participant total mass, including exoskeleton when applicable, and was transformed into joules (i.e., J/kg). Then the Vo2SS was subtracted by the Vo2REST and normalized by the walking speed (di Prampero, 1986).
Data analysis
Data were analyzed using a nonparametric analysis of Longitudinal Data (nparLD) (package nparLD, version 2.1, R software; (Noguchi et al., 2012) ). As there were only six participants in two different groups, data were analyzed as single case datasets via six separate repeated measures analysis (i.e., one per participant) assigning the speed the role of "subjects" within the statistical model. The change in the MCW was the dependent variable and as the independent variable, three conditions were compared with data from the randomly ordered speeds used as the source of variance. As a rank-based analysis of variance, the nparLD procedure is robust to small sample size and produces effect size estimations, named relative treatment effects (RTE) that will range between 0 and 1 and 0.5 as the null hypothesis. The nparLD derives an ANOVA-type statistic (ATS) that provides a robust estimate of differences within repeated discrete data that is not dependent on distribution or variability.
RESULTS
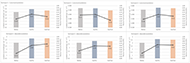
Six healthy participants participated in the study (6 male; age = 30±8; height = 175.2±5.6 cm; weight = 81±7.1 kg). There was a statistically significant effect between conditions for all participants except participant 3 (customized exoskeleton). Overall, the net MCW as well as the RTE shows an increase during the first condition (Exopre) and change depending on the exoskeleton tested. The mean net MCW and RTE are illustrated in Figure 1.
Post-hoc results showed a statistically significant increase effect during ExoPre with the Gen 3.0 and Gen 4.0 for all participants except one (customized exoskeleton) with a relative treatment effect (RTE) of 0.60 to 0.69. Gen 3.0 also reduced their MCW to baseline condition or below in ExoPost condition for two participants while all participants had an increased effect with Gen 4.0. All participants in Gen 4.0 showed no effect of familiarization. Table 1 present the RTE {95% confidence intervals} and p-values of the ATS, between conditions for all participants.
|
Customized exoskeleton (Gen 3.0) |
Adjustable exoskeleton (Gen 4.0) |
||||
Participant 1 |
Participant 4 |
|||||
No Exo |
ExoPre |
ExoPost |
No Exo |
ExoPre |
ExoPost |
|
No Exo |
|
0,60 {0,53; 0,66} ˂ .001 |
0,56 {0,48; 0,63} .102 |
|
0,69 {0,53; 0,74} ˂ .001 |
0,71 {0,56; 0,74} ˂ .001 |
ExoPre |
|
|
0,44 {0,39; 0,49} .0151 |
|
|
0,54{0,43; 0,64} .24 |
|
Participant 2 |
Participant 5 |
||||
No Exo |
|
0,52 {0,43; 0,60} .721 |
0,38 {0,32; 0,47} ˂ .001 |
|
0,64 {0,44; 0,73} .01 |
0,58 {0,47; 0,67} .006 |
ExoPre |
|
|
0,37 {0,32; 0,43} ˂ .001 |
|
|
0,51 {0,34; 0,67} .80 |
|
Participant 3 |
Participant 6 |
||||
No Exo |
|
0,62 {0,48; 0,70} .043 |
0,56 {0,47; 0,64} .192 |
|
0,69 {0,55; 0,74} ˂ .001 |
0,69 {0,58; 0,74} ˂ .001 |
ExoPre |
|
|
0,47 {0,38; 0,56} .289 |
|
|
0,49 {0,32; 0,66} .87 |
DISCUSSION
Part of our hypotheses were supported, as the first exposure to an exoskeleton (custom or adjustable) increased the MCW, but the increase is different between types of exoskeleton. After familiarization, users reduced their MCW when using the customized exoskeleton. However, when using the adjustable exoskeleton, MCW remained the same as ExoPre condition. Literature on that subject is quite clear that metabolic cost is increasing as mass and distance of the load from the participant's centre of mass increase. As the Gen 3.0 weighted around 7 kg and the Gen 4.0 is around 10 kg, this could explain the higher 9% initial increase in MCW with the adjustable exoskeleton.
Interestingly, the familiarization period this not influence the MCW in the same way between the customized and the adjustable exoskeleton. Multiple hypothesis can be generated from these results. First, as the theoretical design and functionality of both exoskeletons are the same, there clearly seems that the adjustment of the exoskeleton on the user has an impact on the user but also on motor learning. Having a 3D representation of the participant would procure a better fit than a visual inspection of joint alignments, which could affect the biomechanics or effective performance as stated in a recent paper [13].
The second hypothesis could be formed into a question: is the device more complex because of added adjustability. As stated in the methods section, the customized exoskeleton was specifically designed using SolidWorks® by using a 3D model of the participant. This means that specific parts were created to adjusts for the circumference of length of a segment. On the other hand, the adjustable exoskeleton requires a change in sizing to adjust on the participant (i.e., thigh length includes parts ranging from 10 mm to 130 mm by 10 mm increment or small, medium, large for thigh circumference). As this is a full body passive exoskeleton, there are a large number of customized parts that need to be changed, which naturally increases the complexity of the device.
CONCLUSION
As an assistive device, customized exoskeleton may be beneficial for reducing MCW and risk of injuries. However, integrating a fully adjustable exoskeleton does not provide the same physiological benefits when walking. A future hybrid exoskeleton should be designed as it could increase the benefit while keeping cost sufficiently low to be accessible to the general population.
REFERENCES
[1] Bertram JE, Ruina A. Multiple walking speed-frequency relations are predicted by constrained optimization. J Theor Biol. 2001 Apr;209(4):445–53.
[2] Donelan JM, Kram R, Kuo AD. Mechanical and metabolic determinants of the preferred step width in human walking. Proceedings Biol Sci. 2001 Oct;268(1480):1985–92.
[3] Liew B, Morris S, Netto K. The Effect of Backpack Carriage on the Biomechanics of Walking: A Systematic Review and Preliminary Meta-Analysis. J Appl Biomech [Internet]. 2016;32(6):614–29. Available from: https://journals.humankinetics.com/view/journals/jab/32/6/article-p614.xml
[4] Seay JF. Biomechanics of Load Carriage--Historical Perspectives and Recent Insights. J strength Cond Res. 2015 Nov;29 Suppl 1:S129-33.
[5] Lowe BD, Billotte WG, Peterson DR. ASTM F48 Formation and Standards for Industrial Exoskeletons and Exosuits. IISE Trans Occup Ergon Hum Factors [Internet]. 2019;7(3–4):230–6. Available from: https://doi.org/10.1080/24725838.2019.1579769
[6] Panizzolo FA, Galiana I, Asbeck AT, Siviy C, Schmidt K, Holt KG, et al. A biologically-inspired multi-joint soft exosuit that can reduce the energy cost of loaded walking. J Neuroeng Rehabil. 2016 May;13(1):43.
[7] Diamond-Ouellette G, Telonio A, Karakolis T, Leblond J, Bouyer L, Best KL. Investigating the change in metabolic cost of walking before and after familiarization with a passive load-bearing exoskeleton: A case series. IISE Trans Occup Ergon Hum Factors. 2022;
[8] Lee S, Kim J, Baker L, Long A, Karavas N, Menard N, et al. Autonomous multi-joint soft exosuit with augmentation-power-based control parameter tuning reduces energy cost of loaded walking. J Neuroeng Rehabil. 2018 Jul;15(1):66.
[9] Gregorczyk KN, Hasselquist L, Schiffman JM, Bensel CK, Obusek JP, Gutekunst DJ. Effects of a lower-body exoskeleton device on metabolic cost and gait biomechanics during load carriage. Ergonomics. 2010;53(10):1263–75.
[10] van Dijk W, van der Kooij H, Hekman E. A passive exoskeleton with artificial tendons: design and experimental evaluation. IEEE Int Conf Rehabil Robot. 2011;2011:5975470.
[11] Burnett AF, Cornelius MW, Dankaerts W, O'sullivan PB. Spinal kinematics and trunk muscle activity in cyclists: a comparison between healthy controls and non-specific chronic low back pain subjects-a pilot investigation. Man Ther. 2004 Nov;9(4):211–9.
[12] Poggensee KL, Collins SH. How adaptation, training, and customization contribute to benefits from exoskeleton assistance. Sci Robot [Internet]. 2021 Sep 29;6(58):eabf1078. Available from: https://doi.org/10.1126/scirobotics.abf1078
[13] Stirling L, Kelty-Stephen D, Fineman R, Jones MLH, Daniel Park BK, Reed MP, et al. Static, Dynamic, and Cognitive Fit of Exosystems for the Human Operator. Hum Factors [Internet]. 2020; Available from: https://doi.org/10.1177/0018720819896898